How AI Labeling Grew From Cats and Dogs to Cancer Cells
When people think of AI training data, the first thing that often comes to mind is the classic cat vs. dog image classification task. It’s a meme-worthy example that’s been around since the early days of machine learning and honestly, it’s not too far from the truth. For years, public image datasets made up of household pets and vacation photos were the foundation for testing and improving computer vision models. But the world of data labeling has come a long way since then.
As AI has moved from academic labs into real-world applications, the need for more precise, diverse, and high-quality labeled data has exploded. What started with teaching models to tell apart a tabby from a terrier has now evolved into building systems that can spot early signs of cancer in medical scans, detect threats in cybersecurity logs, and guide autonomous vehicles through unpredictable environments.
Let’s talk about how we got here and why human-led data labeling still matters more than ever.
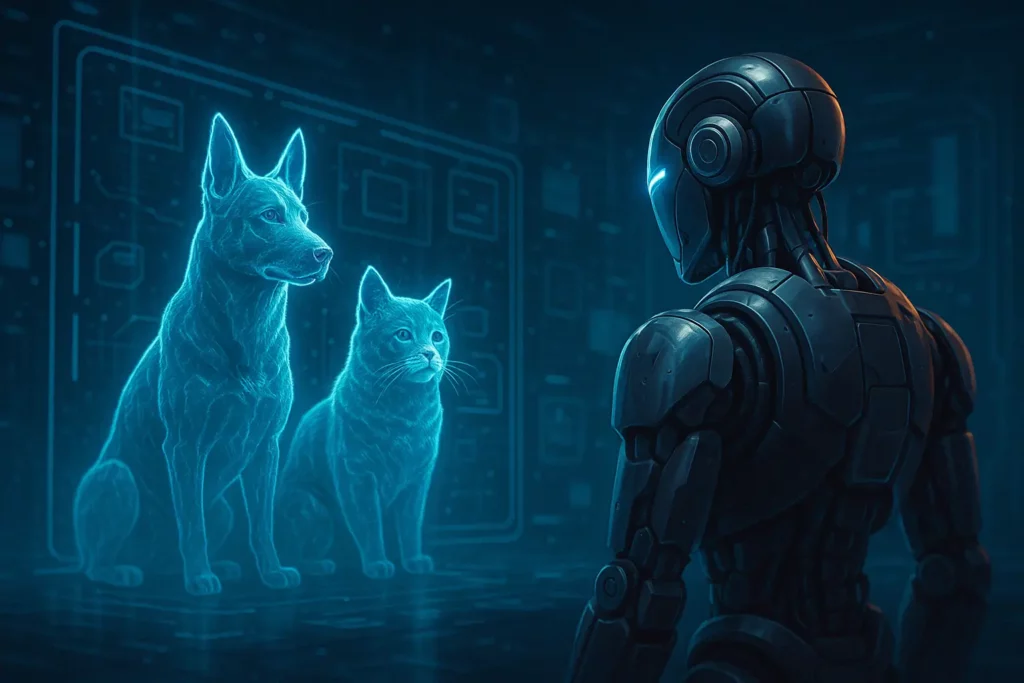
Early Days: Internet Images and Basic Labels
In the beginning, most machine learning models were trained on large but simple datasets like ImageNet or CIFAR. These collections were often scraped from the internet and labeled with basic categories: “car,” “dog,” “banana,” and “chair.” Volunteers or crowdsourced workers mostly did the labeling with limited context or quality checks.
The results were decent for general classification tasks. But these labels were shallow. A photo of a dog was just labeled “dog,” with no extra information about the breed, angle, environment, or quality of the image. That was fine for basic model training, but the moment you tried to apply those models to anything complex; they fell short.
The Shift to High-Stakes AI
As AI tools entered industries like healthcare, finance, security, and transportation, the bar for labeling quality skyrocketed.
In medical imaging, for example, it’s not enough to label an x-ray “healthy” or “not healthy.” Annotators now must outline the exact borders of a tumor, distinguish between different types of tissue, and sometimes even label according to strict clinical guidelines. And they’re doing it across thousands of images, with accuracy that directly impacts patient care.
In autonomous driving, images from vehicle cameras are labeled with extreme detail – every lane, every pedestrian, every shadow that could confuse a sensor. It’s no longer just about recognizing a stop sign. It’s about knowing whether that sign is partially blocked, covered in graffiti, or distorted by sunlight.
More Data, More Complexity, More Risk
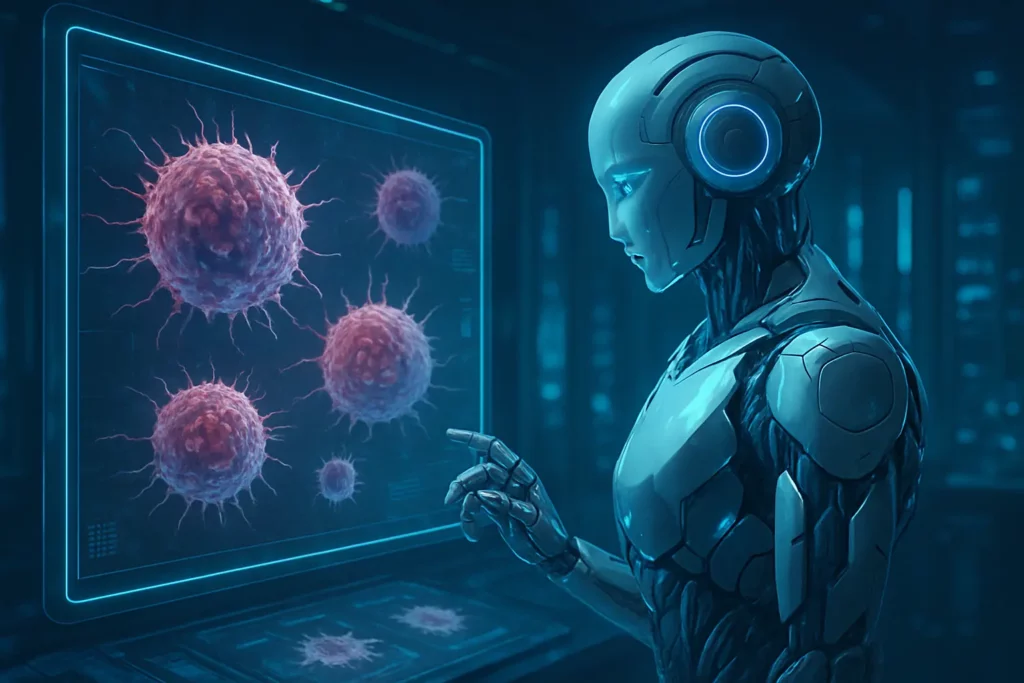
The more complex the data, the higher the risk of error. One missed label in a cybersecurity dataset could mean a vulnerability goes unnoticed. One incorrect medical annotation could train a model to misdiagnose.
This is why the human side of labeling hasn’t gone away. It’s become even more essential.
At Thoth AI, we work with skilled annotators who understand the domains they’re labeling for. We build feedback loops with clients to constantly refine labeling criteria. We QA everything because, in high-stakes environments, labeling can’t be an afterthought.
What’s Next
AI labeling is now less about sheer volume and more about precision, domain knowledge, and traceability. We’ve gone from cute internet animals to cancer cells, legal transcripts, and battlefield simulations. It’s clear that the future of AI depends on how well we continue to label the present.
Because, at the end of the day, a model is only as smart as the data it’s trained on. And that data still needs a human touch.