Enhancing AI Capabilities with Optimized Retrieval-Augmented Generation (RAG)
It is no secret that AI has been evolving rapidly, so using cutting-edge techniques to improve system performance is crucial. One such technique is Retrieval-Augmented Generation (RAG), which is a hybrid approach that combines the strengths of retrieval-based systems and generative models. If organizations incorporate RAG optimization strategies, they can enhance AI capabilities, which will help in delivering more accurate, reliable, and contextually relevant responses across various applications.
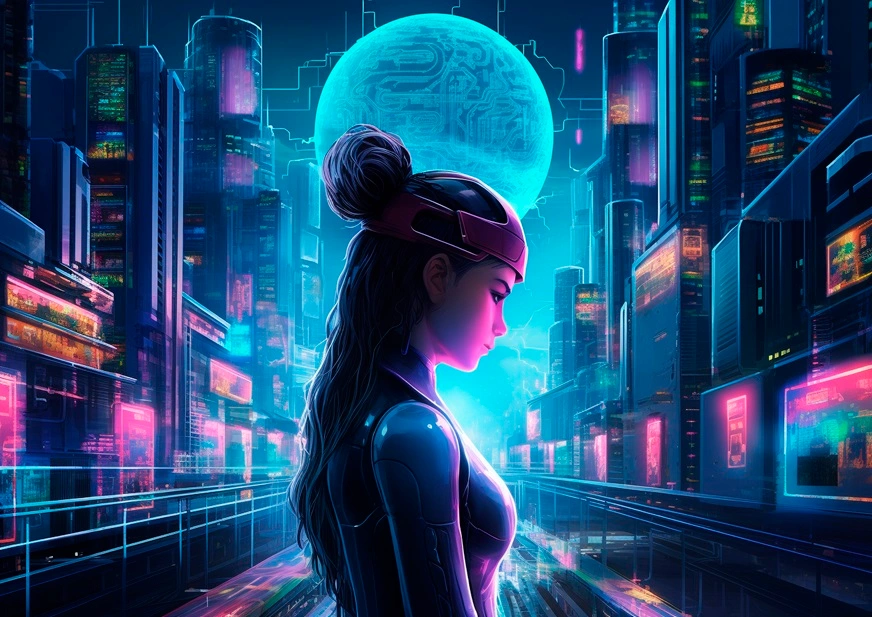
What is Retrieval-Augmented Generation (RAG)?
RAG is an AI framework that works by getting relevant information from external data sources and using it to generate responses through a language model. This approach merges the best of both worlds: the accuracy of retrieval-based methods and the creativity and flexibility of generative AI.
Instead of relying only on pre-trained language models before generating responses, RAG pulls large amounts of structured or unstructured data. This lets AI systems produce accurate answers, especially when dealing with niche or specialized queries.
The Role of Data Enhancement in RAG Optimization
The quality of the retrieved data is critical for RAG to perform effectively. Data enhancement strategies play a pivotal role in optimizing RAG models, ensuring that AI systems are not only retrieving the right information but also leveraging it to improve response generation.
Look at a few data enhancement methods that boost RAG performance:
Data Preprocessing and Cleaning
Ensuring that the data being used is well-organized and free of irrelevant or incorrect information allows the model to focus on retrieving accurate and relevant data, which significantly enhances the quality of the generated responses.
Domain-Specific Data Integration
Incorporating domain-specific datasets into the retrieval process helps fine-tune the model’s understanding and response accuracy within specialized fields, such as healthcare, finance, or legal services.
Metadata Utilization
Leveraging metadata can help the RAG model identify the most relevant content faster. By tagging data with key attributes like time, location, or context, the retrieval process becomes more efficient, enabling the precise generation of answers.
Contextual Embeddings
Boosting RAG with advanced embedding techniques helps the model better understand the context by linking related concepts across different pieces of information, which can drastically improve the quality and coherence of generated responses.
Advantages of RAG Optimization
Optimizing RAG leads to a host of benefits that can significantly enhance AI-powered systems across different industries:
- Improved Response Accuracy: With optimized data retrieval and enhanced context understanding, RAG models produce responses that are more accurate, reducing errors and irrelevant answers.
- Scalability Across Use Cases: Whether applied to chatbots, document summarization, or customer service, RAG optimization helps systems adapt to various use cases by pulling relevant information from a wide range of datasets.
- Reduced Model Size Requirements: By using retrieval-based techniques, RAG models can focus on generating responses based on specific pieces of information rather than requiring vast amounts of pre-trained data. This results in a more efficient and potentially smaller model.
- Enhanced Real-Time Capabilities: In dynamic environments where real-time information is crucial, such as news services or financial markets, optimized RAG models excel at retrieving and using up-to-date information, ensuring the AI’s responses are timely and relevant.
Best Practices for Optimizing RAG
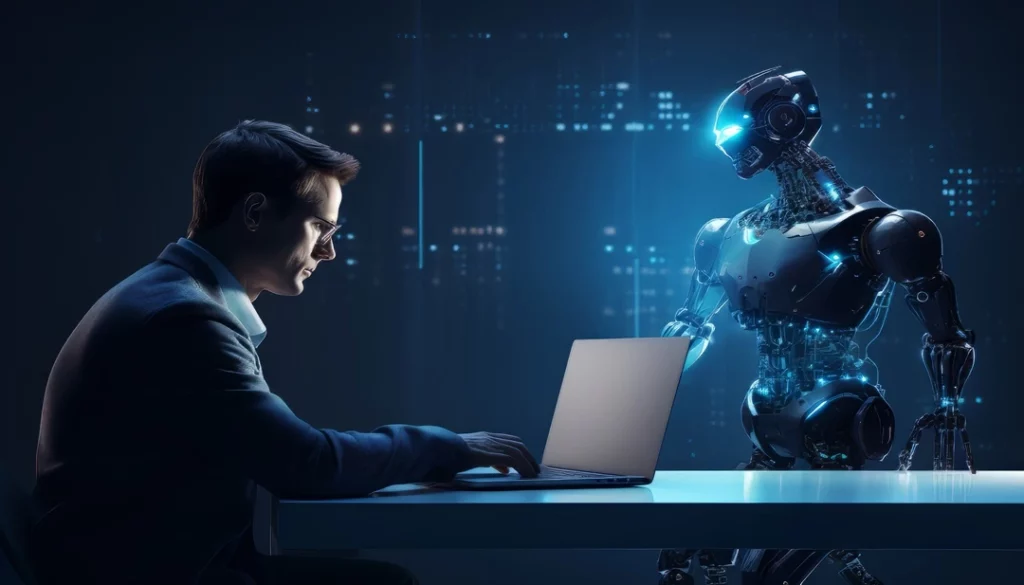
To fully unlock the potential of RAG, there are several best practices for optimizing its performance:
- Continuous Data Enrichment
Continuously integrating fresh, high-quality data keeps the retrieval system updated with the latest information, ensuring that the AI stays relevant and responsive to evolving topics.
- Model Fine-Tuning
Regularly fine-tuning both the retrieval and generative components of the model allows businesses to customize their AI systems to specific tasks or industries, further enhancing accuracy and performance.
- User Feedback Loops
Incorporating user feedback into the optimization process enables iterative improvements. By analyzing which responses were useful or accurate, the system can fine-tune its retrieval and generation capabilities.
Conclusion
Improving Retrieval-Augmented Generation (RAG) presents a significant opportunity for organizations to streamline their AI performance. By focusing on data quality, context relevance, and continuous improvements, businesses can create AI systems that deliver precise, timely, and context-aware responses. As industries continue to demand more from AI-powered applications, RAG optimization stands out as a critical tool for unlocking new levels of success and efficiency.